My main fields are machine learning and artificial intelligence in
computer science. This encompasses a broad spectrum of research areas and
applications, aiming to develop intelligent systems that can learn from
data, make decisions, and perform tasks autonomously.
Within artificial intelligence, I specialize in several cutting-edge
areas, including brain-inspired learning, hyperdimensional computing,
neuro-symbolic AI, hardware-algorithm co-design of AI models,
interpretable and transparent AI, and intelligent sensing.
For more information on my research, visit this link.
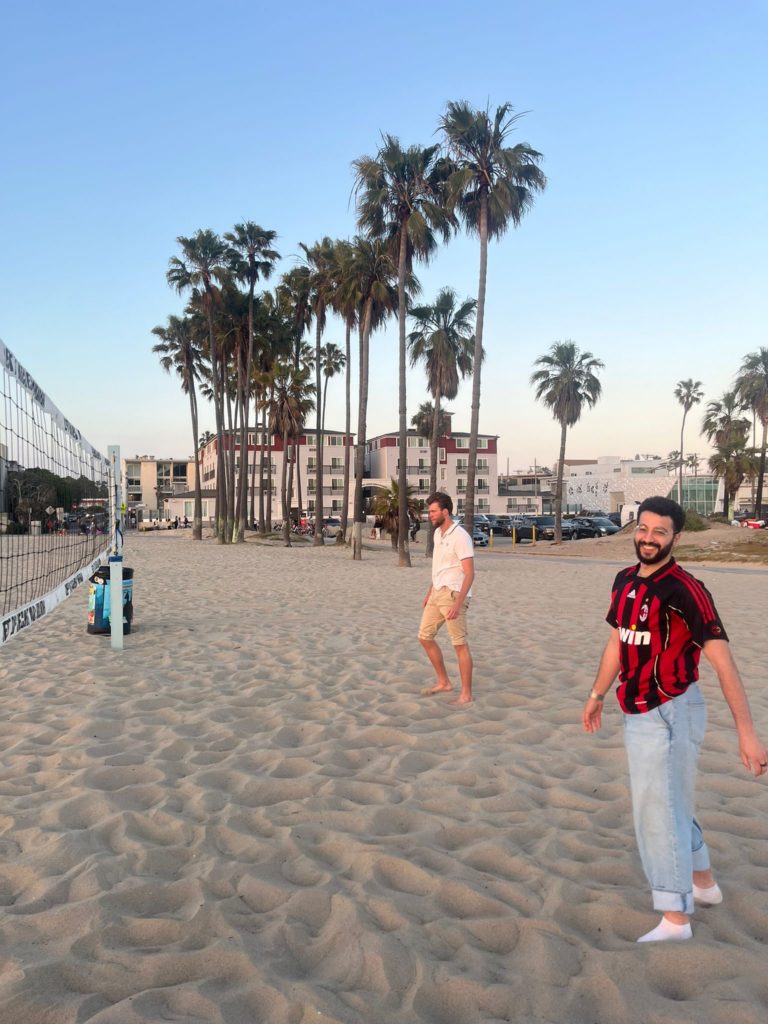
My Unique Position in the Field
My research addresses the significant challenges of transparency and understanding in machine learning models.
While traditional ML has advanced rapidly, the need for interpretable and trustworthy AI remains critical,
especially in applications like healthcare and finance. Neurosymbolic AI and brain-inspired learning represent
the future of AI, combining neural networks and symbolic reasoning to create robust, adaptable,
and understandable systems. These fields, though complex and novel, hold immense potential for achieving
artificial general intelligence.
Working under Dr. Mohsen Imani, a leading researcher in this field, and leading multiple projects in his lab,
has given me a distinctive edge. My expertise in Fourier analysis, gained from my undergraduate degree in
Electrical Engineering, allows me to approach AI problems uniquely.
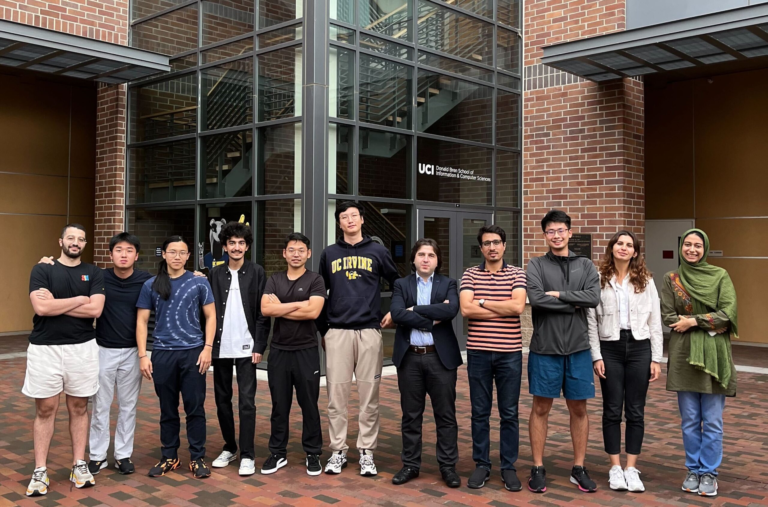
Key Research Contributions
My research contributions include adapting hyperdimensional computing algorithms for computing in-memory
technologies based on FeFET or Photonics for many applications where these emerging technologies are highly
needed, such as genetics, biology, or reasoning. I have developed methods to address complex tasks like
reasoning on edge and resource-constrained devices, enhancing computational and energy efficiency.
Additionally, I have conducted deep studies on the safety of these new systems and contributed theoretical
advancements, ensuring robust and efficient AI systems.
Future Endeavors
My goal is to develop interpretable, transparent, and robust AI methodologies, particularly in brain-inspired
learning, neurosymbolic AI, and hyperdimensional computing. These approaches aim to foster a trustworthy
relationship between AI systems and society.
I plan to leverage new, imperfect, and noisy technologies to bring numerous advantages to applications such as
genome sequence matching, graph reasoning, and object detection. By utilizing advanced mathematical modeling
and AI algorithm development, I aim to fundamentally change how information is encoded in HDC systems to handle
noise and non-idealities.
I am pioneering near-sensor AI research, where AI computations occur close to the data source, reducing the
need for centralized processing. This approach is vital for environments where data is constantly generated but
only occasionally of interest, such as security systems and healthcare devices.
My research is already recognized through my publications in prestigious conferences and journals like DATE,
IEEE TCAS 1, ICCAD, and Nature Scientific Reports, with over 85 citations as of May 23, 2024. I currently have
several papers awaiting acceptance, including one submitted to NeurIPS. I will continue to share my work
through peer-reviewed publications, conference presentations, and collaborations with leading researchers,
ensuring ongoing engagement and impact.
You can find my Google Scholar profile here: Link