Welcome to my research portfolio. I am Hamza Errahmouni Barkam, a dedicated PhD researcher at BiasLab,
UC Irvine, focusing on cutting-edge AI methodologies.
My work revolves around making artificial intelligence more interpretable, transparent, and robust.
I specialize in brain-inspired learning, neurosymbolic AI, and hyperdimensional computing.
Through my research, I aim to bridge the gap between advanced AI technologies and their practical
applications in various domains, particularly healthcare, security, and edge computing.
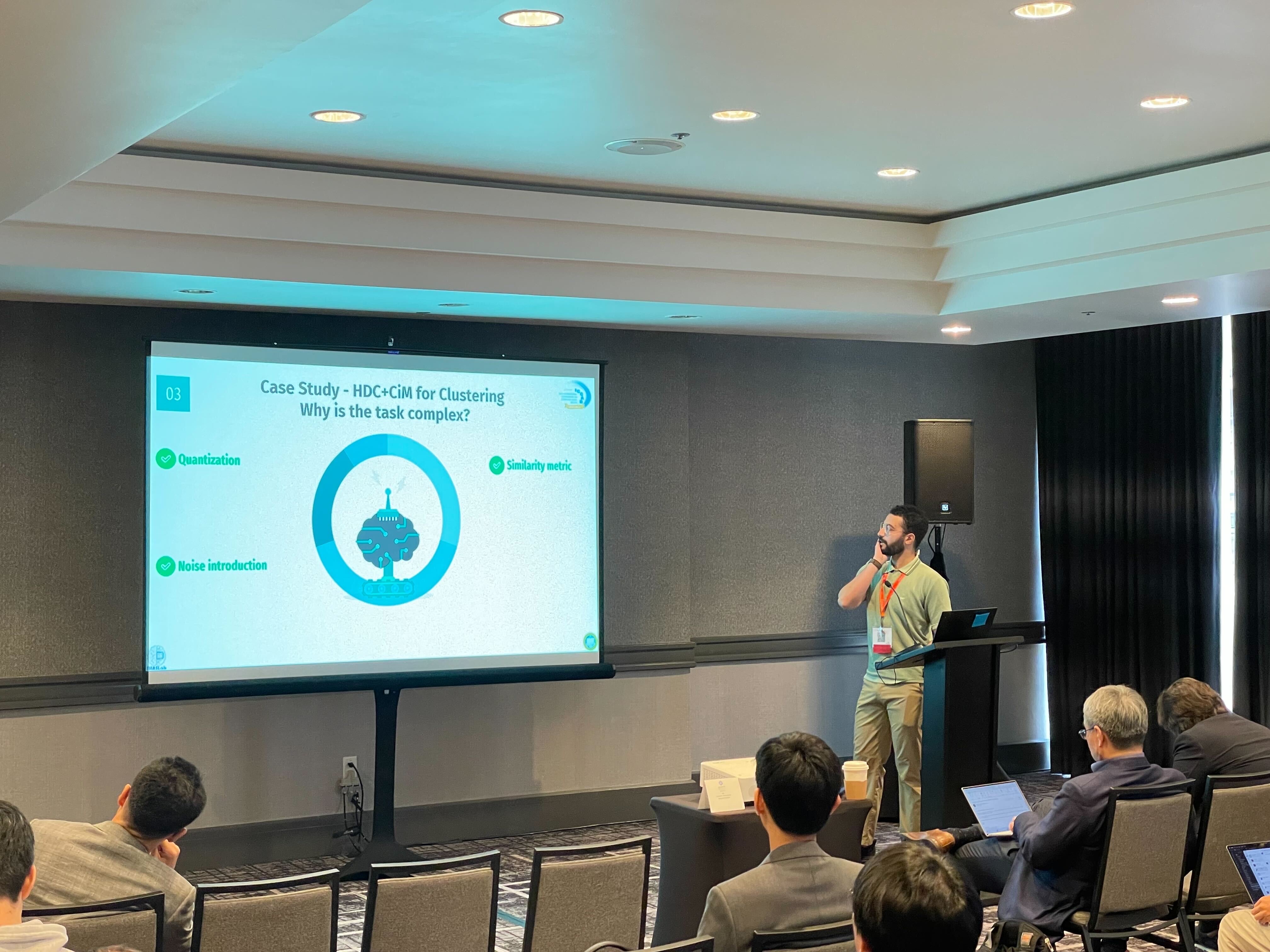
Purpose of my research
Machine Learning and Artificial Intelligence
Machine Learning (ML) and Artificial Intelligence (AI) are at the heart of my research and represent
transformative technologies reshaping our world. AI involves creating systems that mimic human
intelligence, enabling machines to perform complex tasks such as language comprehension, pattern
recognition, and decision-making. Within AI, Machine Learning focuses on developing algorithms
that empower computers to learn from data and improve their performance autonomously.
By harnessing the power of data and advanced computational techniques, ML models continuously
evolve and enhance their capabilities without explicit programming. My work leverages these
cutting-edge technologies to push the boundaries of what is possible, driving innovation and
efficiency across healthcare, security, and beyond.
Issues with traditional Machine Learning
Traditional Machine Learning (ML) has achieved remarkable successes, but it faces significant
challenges that limit its applicability and reliability, particularly in critical fields like
healthcare and security. One of the most pressing issues is the "black box" nature of many ML models,
especially deep neural networks. These models often operate without providing clear insights into
their decision-making processes, making it difficult for users to understand or trust their outputs.
This lack of interpretability and explainability poses serious concerns, particularly when AI systems
are used in life-or-death scenarios or when they
influence significant decisions.
Furthermore, while neural networks have demonstrated powerful capabilities, they may not be the best
path toward achieving Artificial General Intelligence (AGI). AGI requires not just specialized, narrow
intelligence but broad, adaptable, and understandable cognitive abilities akin to human reasoning.
In contrast, brain-inspired learning, vector symbolic architectures (VSA), and neurosymbolic AI offer
promising alternatives. Brain-inspired learning models emulate the efficiency and parallelism of the
human brain, providing robust and low-cost solutions that are more aligned with natural intelligence.
Vector symbolic architectures allow for the encoding of complex information in a highly interpretable
and structured manner, facilitating clearer insights into the operations of AI systems. Neurosymbolic
AI combines the strengths of neural networks and symbolic reasoning, enabling systems to reason and
learn in a way that is both powerful and understandable.
These innovative approaches address the critical issues of interpretability and explainability, paving
the way for more transparent, trustworthy, and adaptable AI systems. By focusing on these advanced
methodologies, my research aims to overcome the limitations of traditional ML and advance the field
towards more reliable and human-aligned artificial intelligence.
Neuro-inspired AI and Symbolic AI
Neuro-inspired AI and Symbolic AI represent two innovative approaches to advancing artificial
intelligence. Neuro-inspired AI emulates the human brain's architecture and learning processes,
achieving high performance with lower computational costs and energy consumption. This approach
enhances the robustness and adaptability of AI systems, making them suitable for real-time and
resource-constrained applications.
On the other hand, Symbolic AI uses explicit, human-readable symbols and rules for knowledge
representation and reasoning, providing clear and interpretable decision-making pathways.
Combining these approaches can create powerful, transparent, and trustworthy AI systems that
leverage the strengths of both neural structures and logical reasoning.
Brain-Inspired Learning
Brain-inspired learning models are designed to mimic the structure and functionality of the human brain,
leveraging its remarkable efficiency, parallel processing, and adaptability. By emulating neural
mechanisms, these models achieve high performance with lower energy consumption and computational
costs. Brain-inspired learning offers a robust and scalable approach to developing AI systems capable
of real-time learning and adaptation. This approach is particularly valuable for applications
requiring continuous learning and decision-making in dynamic environments, such as healthcare,
security, and autonomous systems. By drawing from the principles of how the brain processes
information, brain-inspired learning paves the way for more intelligent, efficient, and resilient AI
technologies.
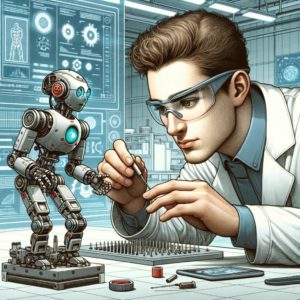
Hyperdimensional Computing
Hyperdimensional Computing (HDC) is an innovative approach that leverages high-dimensional vectors to
represent and manipulate information inspired by the brain's cognitive processes. Unlike traditional
computing paradigms, which often struggle with noise and variability, HDC excels in handling
imprecise and incomplete data. This robustness makes it ideal for applications in real-time and
resource-constrained environments. HDC encodes information into high-dimensional vectors that
can be efficiently processed and stored, enabling fast and parallel computations.
This method enhances the scalability and adaptability of AI systems, offering a promising pathway for
developing resilient and efficient AI solutions. By integrating HDC into various applications, we can
achieve significant improvements in areas such as intelligent sensor data processing, real-time
learning, and adaptive decision-making.
Facebook
Twitter
LinkedIn